Learning From Machine Learning, on designer trees and architectural historiographies of the digital
What does it mean for scholars to collaborate with contemporary knowledge machines? In this article, Sylvia Lavin reflects on the failures, successes, and potentialities of a machine learning tool designed to identify trees in architectural drawings. This project, which she initiated in 2022, was undertaken by Princeton University and the CCA and with the participation of Drawing Matter, and was supported by the Princeton University Humanities Council Magic Grant. This article is published in conjunction with the CCA.
The matter of computation in architecture is the subject of an increasingly robust set of scholarly literatures: over the past ten years, this body of work has extended the interests of the 1990s that theorized digital form making and then focused on fabrication and construction into more specifically historical concerns for the impact of the digital on global developments in architecture’s techno-social ecology. Historians of architecture, like all scholars today, are also themselves increasingly subject to the growing dominion of digital research protocols, accelerated by the many digitization projects that developed in response to COVID-related limitations on physical access to archives and libraries. Today, PDF’s, scanning, Google search, and optical character recognition software undergird the research methods of scholars whose interests and ideological positions otherwise differ. Despite this obvious ubiquity, however, and despite the growing strength of the digital humanities on university campuses, the explicit address of architectural historians to digital presence in their own work has largely focused on the increasingly convergent matters of publication and data management. On-line publication platforms and crowd-sourced archiving projects, often one and the same thing, collect and provide access to data as intellectual work but also typically defer the work of information processing to others.
The rapid consolidation of the affordances of computing for architectural history in terms of dissemination and big data need not preclude the field from considering how a more diverse array of digital presences within architectural history might add to the field’s creative ecology. It is in the spirit of that effort that this project, conceived as a collaboration between Princeton University and the Canadian Centre for Architecture, and with the participation of Drawing Matter, developed a machine learning (ML) tool designed to identify trees in architectural drawings.[1] The goal was to experiment with the use of digital tools to disrupt the conventions for organizing and cataloguing drawings in both archives and ‘collections’ and to update the technique of ‘visual interpretation,’ once a central if contested activity of the architectural historian and today a still common though a less and less theorized procedure. While providing material for others to activate resonates with approaches to information characteristic of the early days of the world wide web, to wit the Whole Earth Catalog or Global Tools, deferral has its own ethical limitations rooted in the empirical fact of delay and the unempirical supposition of objectivity. Rather than neutrality, therefore, this project was conceived as an effort to actively remake relations between things in the world.
For this experiment, ‘drawing’ was prosaically and tautologically defined as anything designated as a drawing and available as a digital image file from the CCA and Drawing Matter collections. This choice was made in the context of a turn towards the ‘document’ as a preferred object of study for architectural historians, understood as an artefactual by-product of the critique of the power structures embedded within both the historical category of the authored architectural drawing and of the expertise required to read it.[2] Precisely because of this contamination, however, drawings are deeply and visibly embedded in the ways in which architecture has defined humanness (the need to counteract the human proclivity to be seduced by images and their dissimulations, for example, is built into the profession’s injunction against perspective, while the capacity of the architectural drawing to smuggle autonomous personhood and subjectivity into an otherwise industrially determined set of operations has been often asserted). As a result, unleashing the machinic into the anthropomorphizing domain of drawing seemed a good way of imagining more varied forms of engagement, and indeed collaboration, between scholars and the digital conditions of their work.
Trees, defined like drawings in equally prosaic terms as woody plants, were selected because they have been conscripted into service as epistemic interfaces between humans and non-humans since at least the biblical era; when Eve consumes the tree of knowledge, she triggers the construction of the world in which architecture dwells. As subjects, therefore, trees authorize the assumption that their presence in a drawing does epistemological work, making them vital alibis for a machine-learning project. At the same time, in part because of this tradition that privileges the arboreal register of the world’s life forms, the omnipresence of trees in architectural drawings is so self-evidently native to the representation of the environment that they have rarely been included in the kinds of metadata that enable scholars to navigate the increasingly large number of drawings that constitute an important strata of the field’s archive.[3] Trees are thus paradoxically pervasive and also invisible across architectural drawings, where they do specific kinds of epistemic work that rely on drawing techniques such as calculating proportions, determining the impact of the sun on the earth, and registering the development of soil science and of dimensioned lumber on construction procedures. Most importantly, because their underlying role in the structuring of architectural representation is to naturalize significant epistemic operations, trees have typically disturbed drawing conventions; they often appear in isometric projection, for example, in images that are otherwise drawn in plan, or as perspective protuberances on otherwise flat elevations.
Each of these perversions, paradoxes, and exceptions made it likely that the ML tool would fail to complete the simple task of identifying trees in architectural drawing. Failure was even more predictable given the fact that the number of digitally accessible drawings available through the CCA and Drawing Matter, even when combined, was far smaller by a factor of thousands than the number of control samples typically used as training sets, even when machines are seeking to learn quite straightforward lessons. Certain dimensions of the field are therefore too small to be captured by digital regimes, a fact that seems essential to emphasize for its risks but even more for its potential benefits. Nevertheless, and despite some degree of failure from a computational point of view, the tool generated several instructive results, including the location of trees that I would not have found otherwise.
In my view, the ability for scholars to bypass overdetermining catalogue and subject headings when searching for materials is a net gain to scholarly process. Unlike the quantifiable metrics sought out by the coders, this advantage does not rely on comprehensively accurate results; even a few surprises can be enough to trigger new avenues of research. Similarly, including drawings in the ‘scanning’ procedures scholars engage in when building a research archive for the history of architecture, whether based on the OCR scanning of documents, the manual flipping through and quick read of paperwork, or Google Image searches of photographic records, can only constitute an expansion of scholarly possibility. Another and certainly more interesting result is the way in which both the false negatives and false positives revealed biases, overdeterminations, and potentialities in a still nascent but likely to become more prevalent means of research. The following footnotes constitute a provisional catalogue of these mesconnaisances with an eye towards converting them into corrections, revisions, and additions to the contemporary scholarly toolkit. Finally, in an effort to begin the thought process of what it means to do scholarship in collaboration with our contemporary knowledge machines, some of the taxonomic descriptions were generated by ChatGPT and Google Bard.
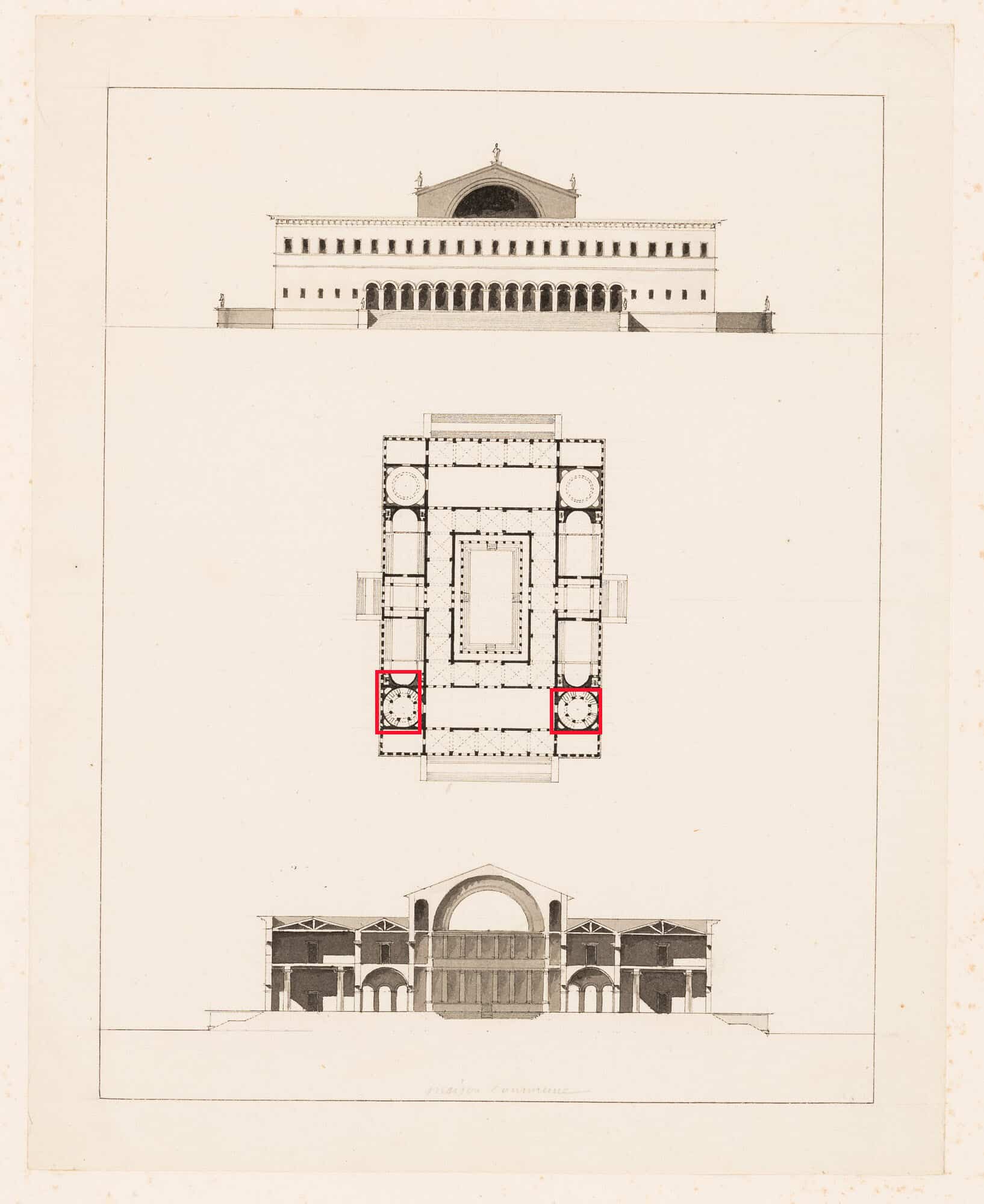
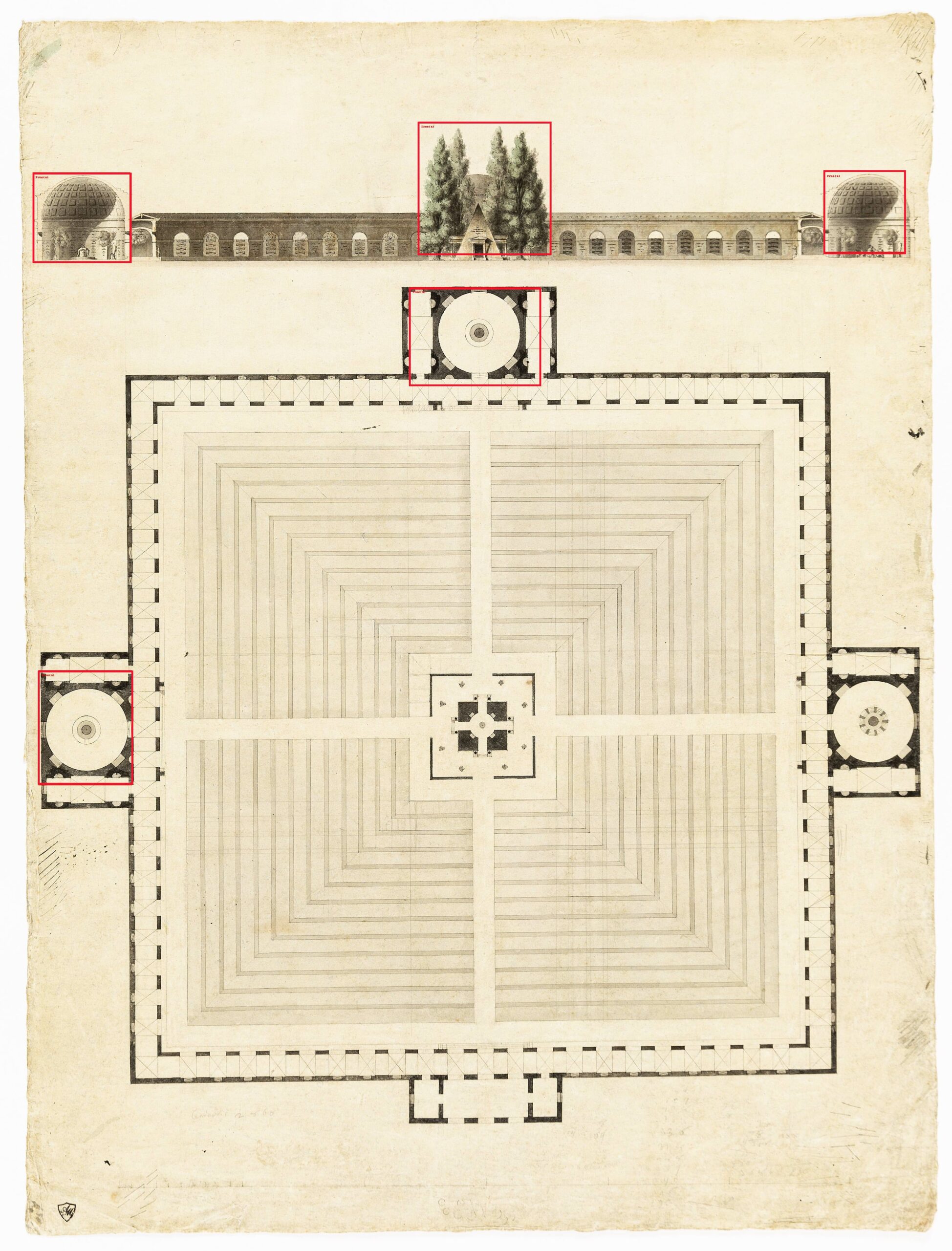
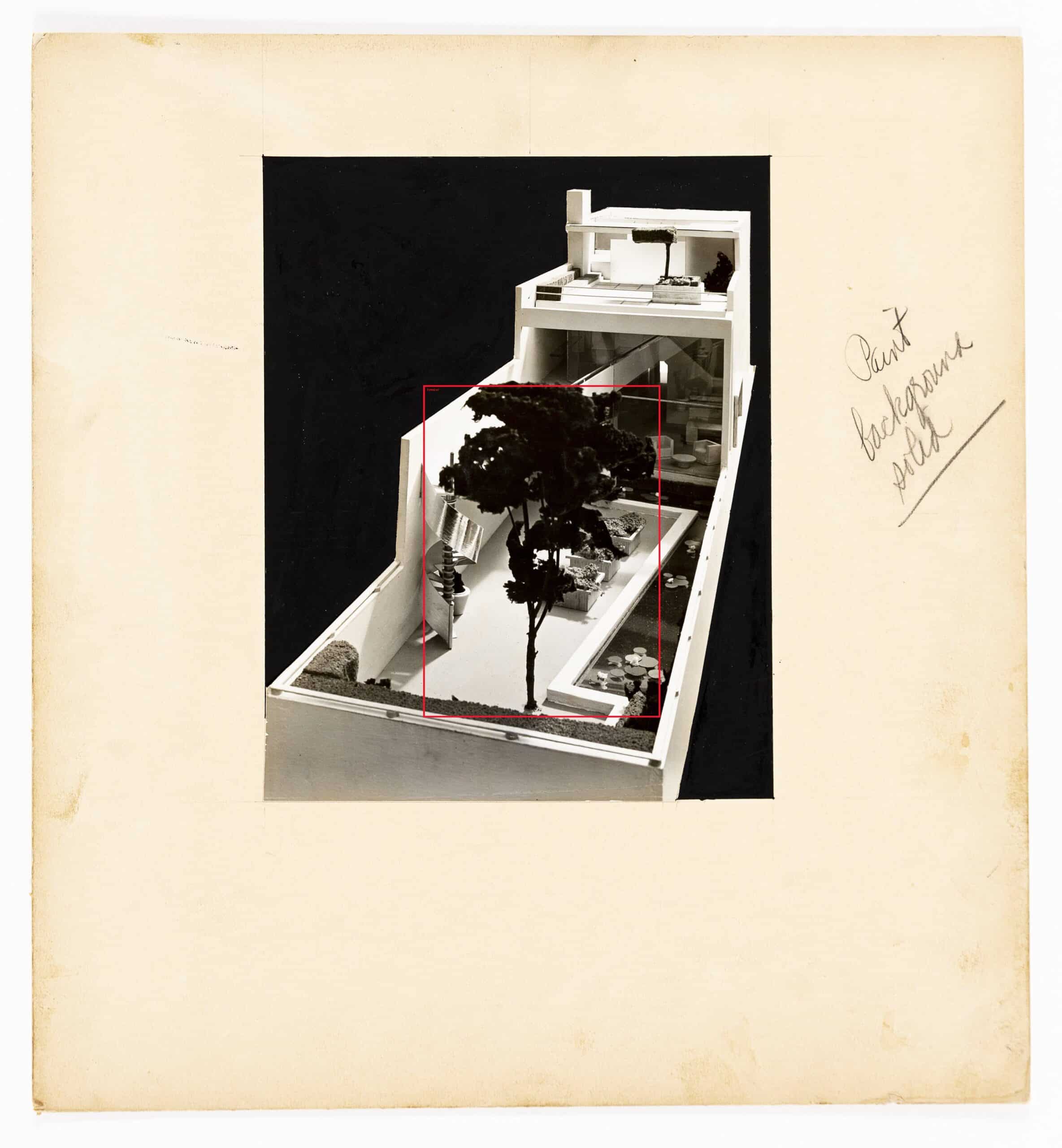
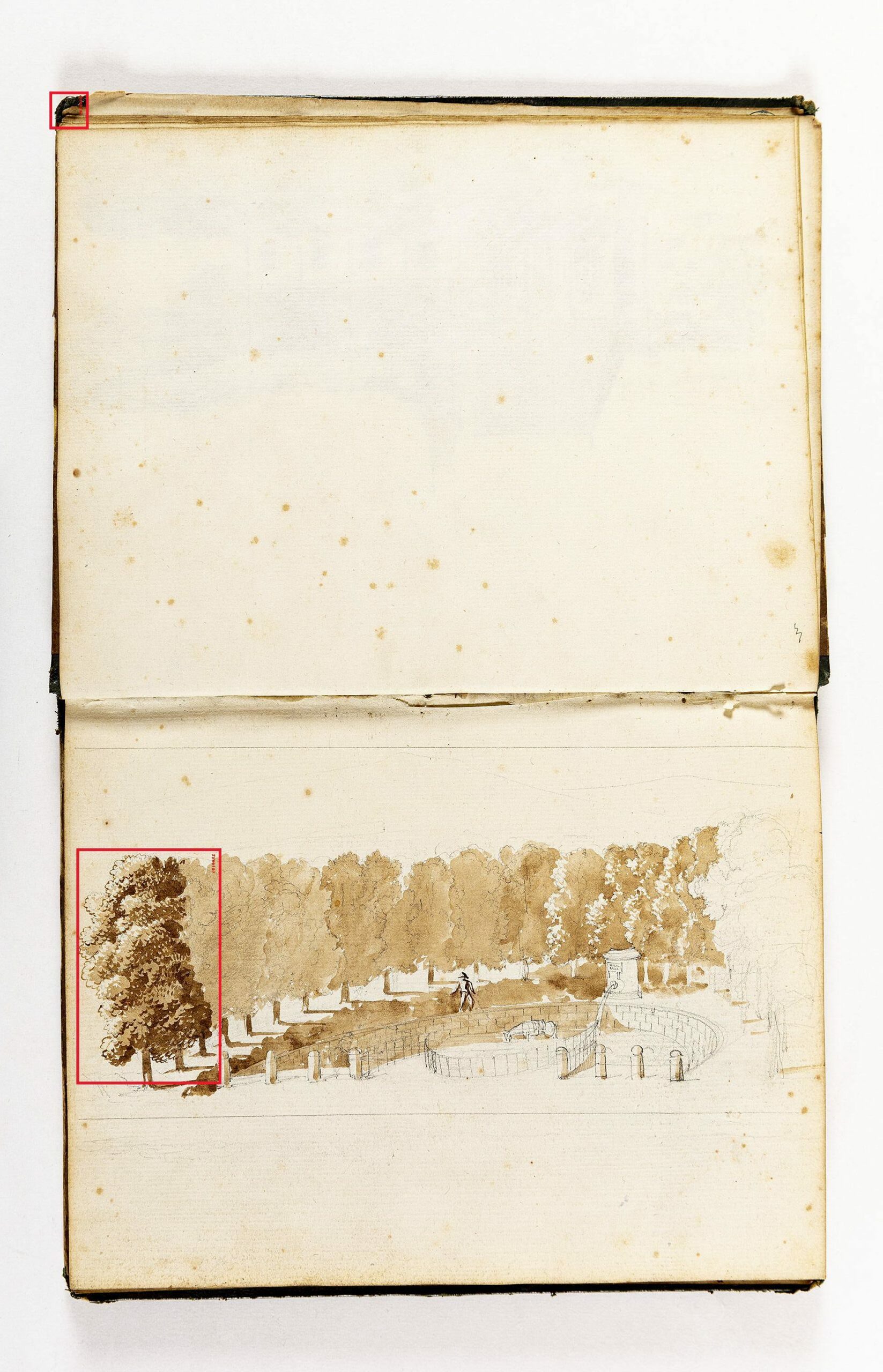
Two category errors were made by the tool consistently enough to suggest an underlying aporia, one in favour of symmetry and one in favour of realism. The interest of these errors lies in what they reveal about the processing that undergirds the results and particularly about distinctions between cognitive/psychological, which is to say, human biases within the algorithmic processes of machine learning and techno-mechanical biases. The bias in favour of symmetry was particularly strong in plans; even if showing an asymmetrical building configuration, the tool planted trees in axially symmetrical arrangements. That the tool did not plant trees to produce symmetry in perspective drawings suggests that the abstraction of the plan invited some form of intervention in the processing; less information encouraged more filling-in the perceived gaps and thus space for projective imagination. This dynamic would also explain the reverse: perspectival and elevational views were accepted at face value, so to speak, and hence did not encourage any actor to fill-in missing information. This is not to say that the tool was accurate when the images were naturalistic, but rather that the processing was less participatory and less presumptive in the face of certain kinds of images.
These observations suggest interesting fissures within the kinds of collaboration between human and nonhuman agents that form machine learning protocols. On the one hand, as is well known, human scriptors bring cultural norms into their work that are deposited into and become part of the otherwise automated system. These ‘contributions’ are especially generous when information is lacking and hence interpolations are if not exactly necessary at least possible.[4] Insofar as architectural plans lack normative forms of naturalistic realism, they mobilize this dynamic: we could say that the corrective impulse revealed, as Claude Perrault would have anticipated, a natural proclivity to seek symmetry on the part of humans, a proclivity that yielded an algorithm dominated by a humorously beaux-arts approach to composition.[5] Because this error is likely the product of cultural biases appearing as symptoms masquerading as technical artefacts, it presents as a new version of an old-fashioned Freudian-slip. The proclivity towards realism, by contrast, appears instead to be the by-product of the fact that machine learning protocols typically depend on very large numbers of photographs. That new research protocols are being developed around old photographic analogs at the moment they are being replaced by new visual logics is worth noting, if only because the slippage between the two is creating a ghost in the machine that generates the impression of an operational unconscious at work.[6] While we typically critique the supposedly surreptitious control of automatic processes by the imposition of human biases and hence politics, the removal of the Freudian slip and other subliminal forms of production seems like a loss worthy of both critique and resistance.
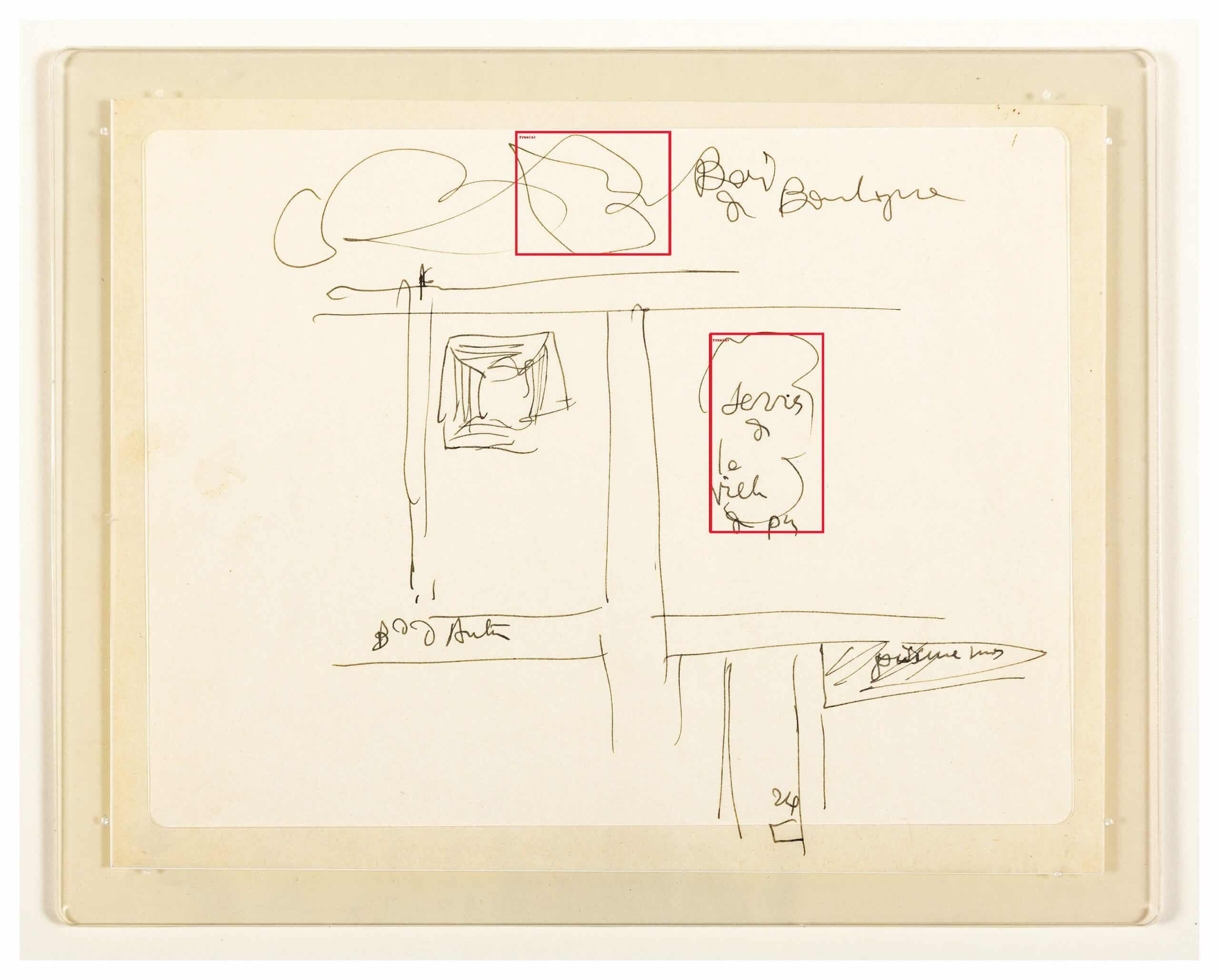
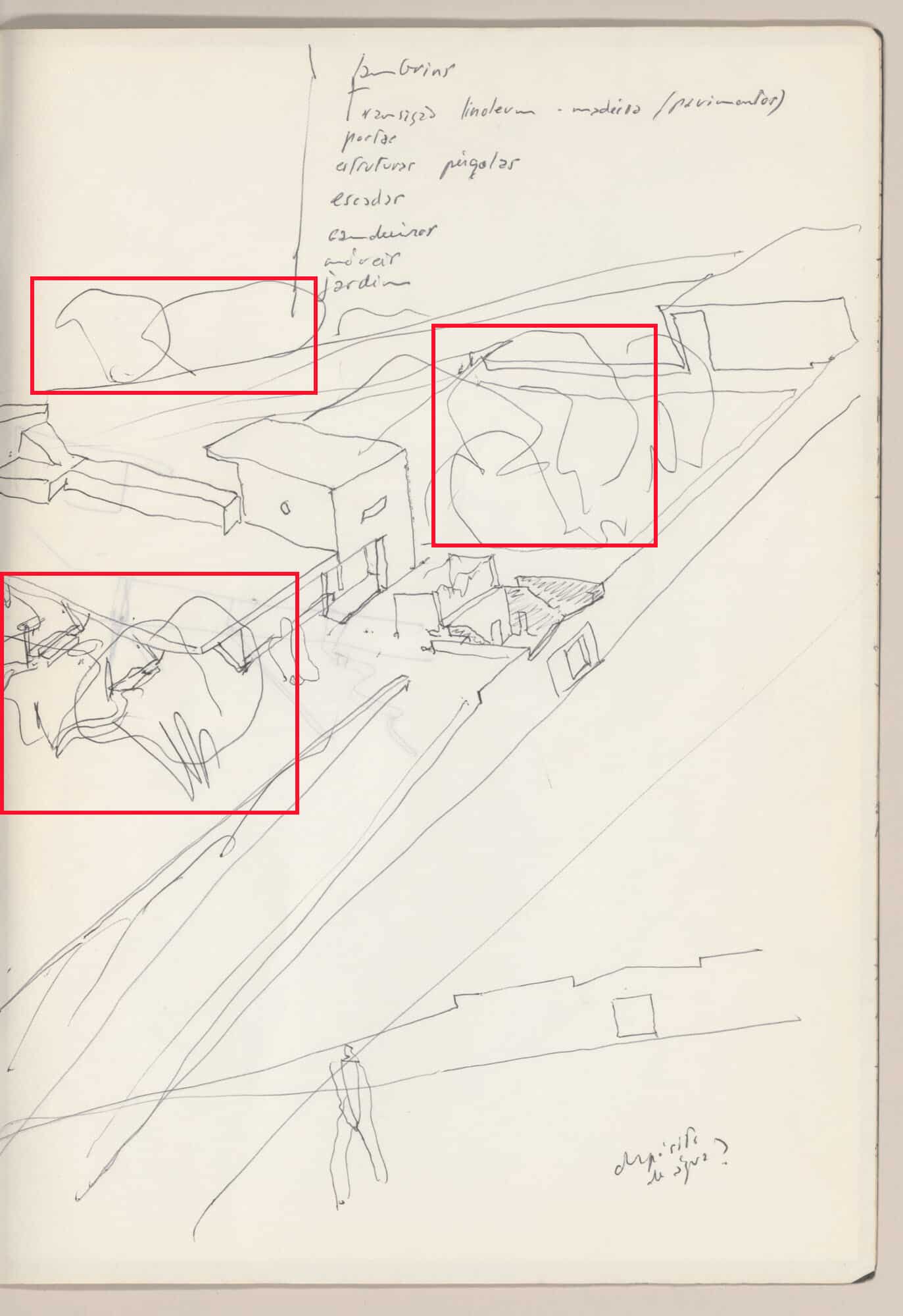
The tool reinforced the dominance of certain architects who have already been identified as important to the history of architecture. Because Le Corbusier and Álvaro Siza have been extensively collected by the CCA and Drawing Matter, the algorithm had more training samples of their drawing idioms than from any other discernably coherent source. As a result of this large data set, the algorithm not only learned to discern trees in their drawings with a comparatively high degree of accuracy, but began to impose that idiom on others as a positive value. If drawings did not show trees à la Siza, the tool was more likely to fail to identify them, converting its failure into a reinscription of Siza’s success. In other words, rather than promoting neutrality with regard to the field’s hero figures and structures of authorship, we are teaching our computers to reinforce those attributes by converting authorial personhood into large sized data sets. The reverse might also be possible: by equalizing inputs, the resulting ‘learning’ curve might be more widely distributed across actors not already over-represented in our collections and catalogues.
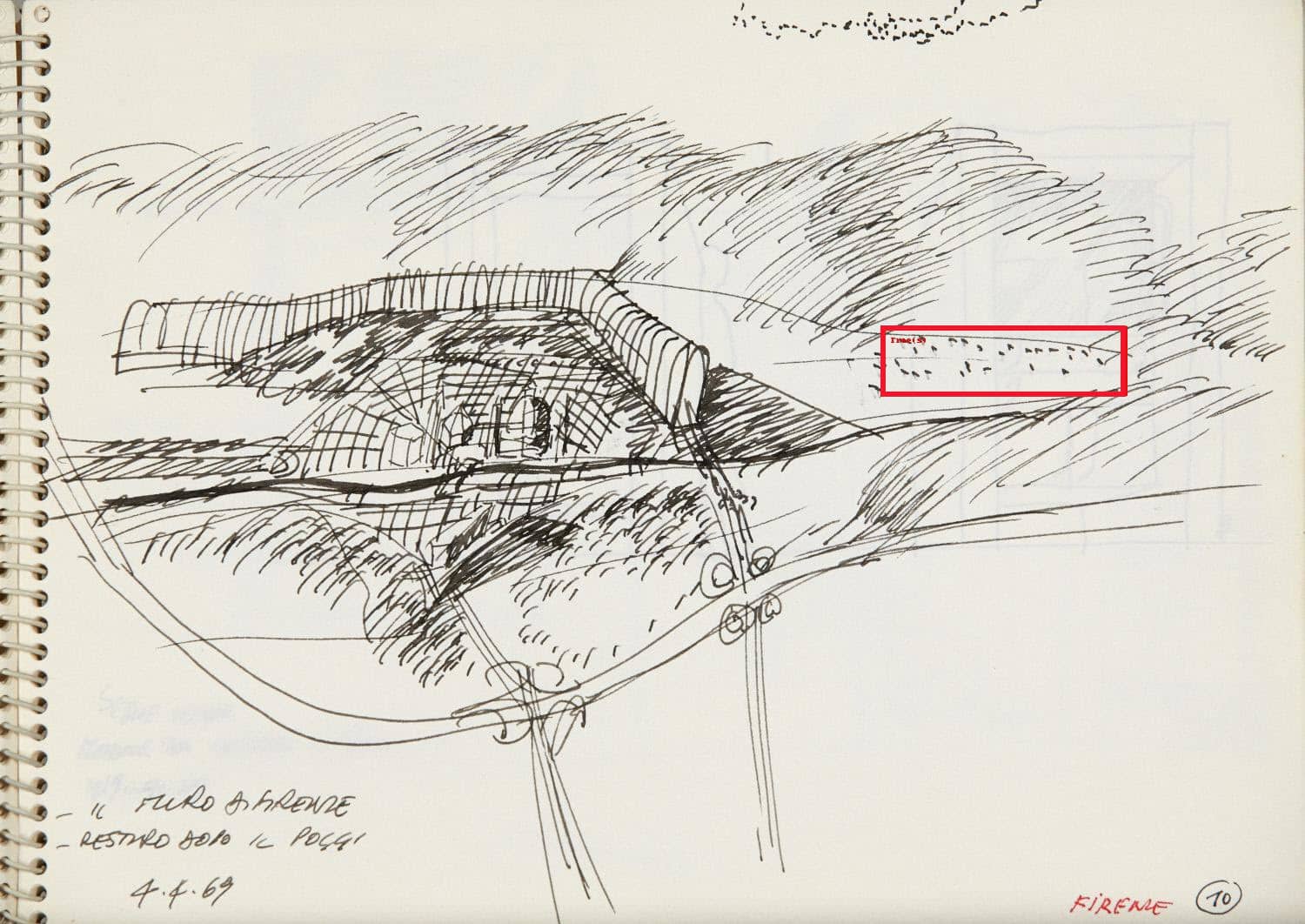
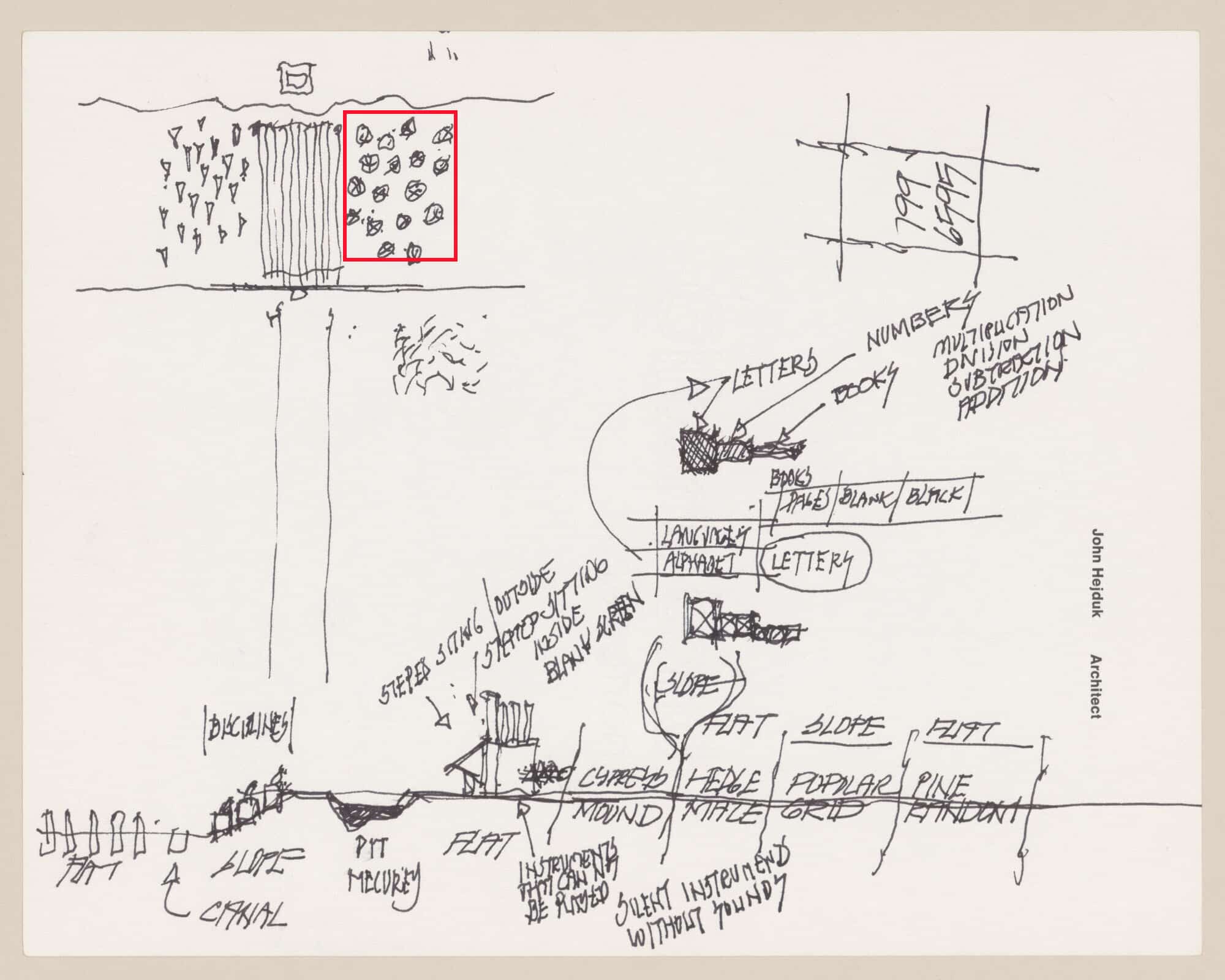
In seeking pixels organized in what it learns to define as arboreal fashion, the tool’s scanning operation is indifferent to how many pixels are needed to constitute any single example as worth learning from. As a result, how big or small the representation of a tree was relative to the size of a drawing as a whole, or whether the tree was drawn in or out of scale with other objects, neither added nor detracted from the tool’s ability to make a determination. In fact, the tool noticed the presence of very small trees, or tiny things it determined to be trees, that I missed. In my view, it is always a good idea to learn how not to be impressed by size.
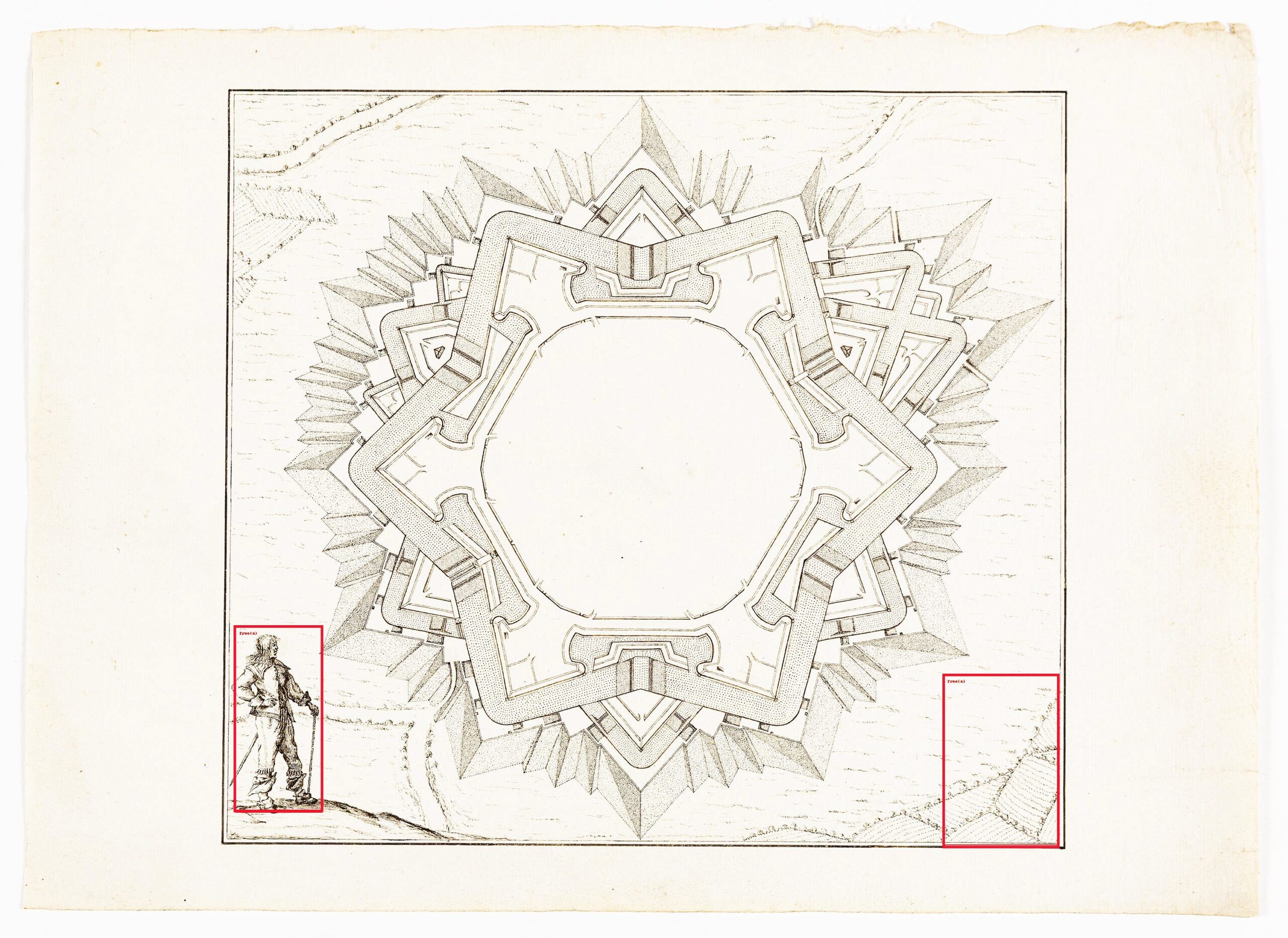
Persons and trees were frequently confused by the tool. According to Google Bard, this can be explained by the fact that ‘machine learning protocols are often trained on data that includes both people and trees. This can lead to the protocol learning to associate certain features with both people and trees, such as the presence of hair or leaves.'[7] Or it could simply be that ML protocols have incorporated the hominizing impetus of most forms of modern exploration and therefore equate the ‘discovery’ of pixels organized in ways that affirm the priority of anthropomorphic shape as an intrinsically positive result. Pointing out that the primitive hut is about valorizing the growing power of the architect and not the trees that are expended in building manufacture, in this context, is less an error or a false positive than it is the product of an accidentally critical hermeneutics.
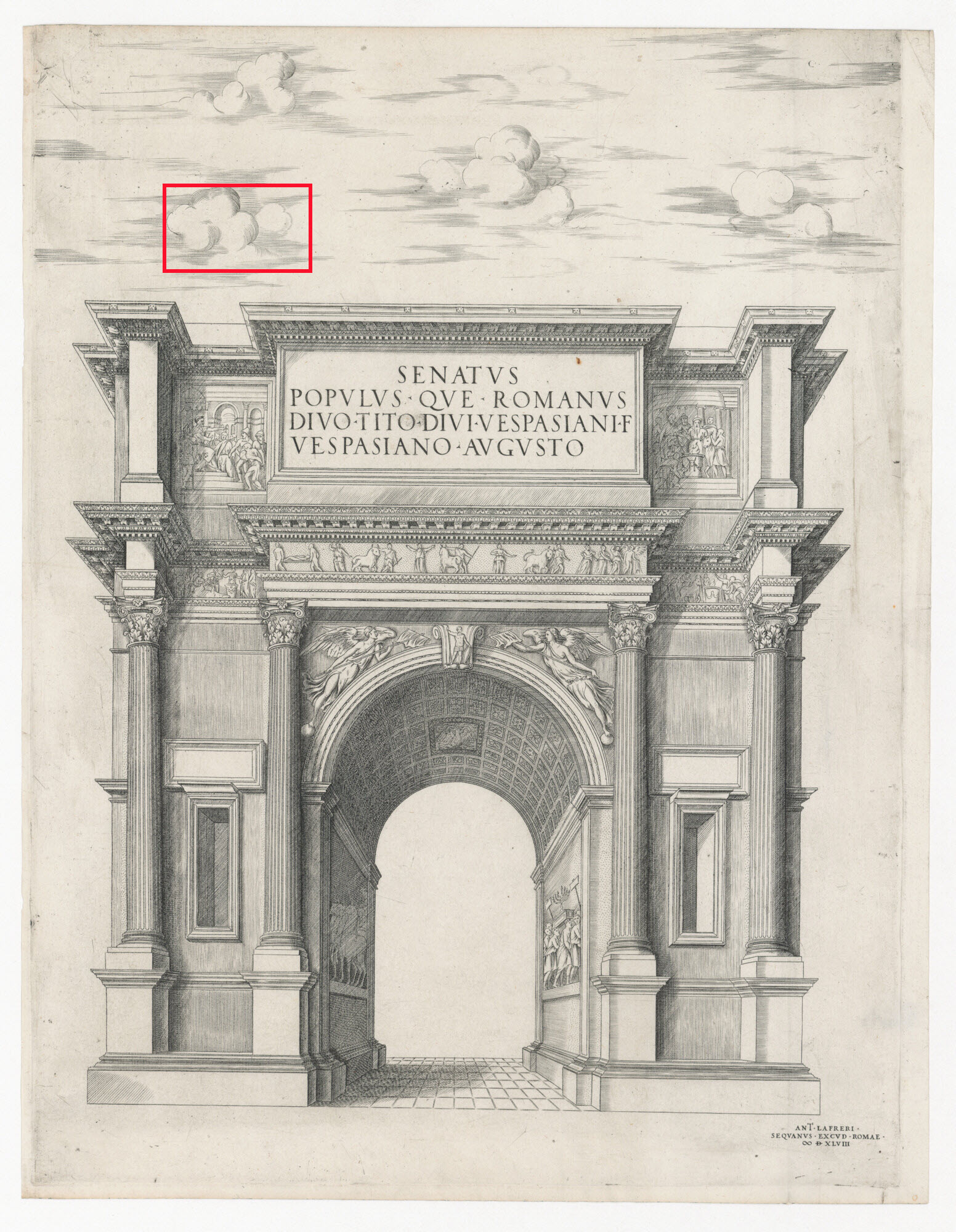
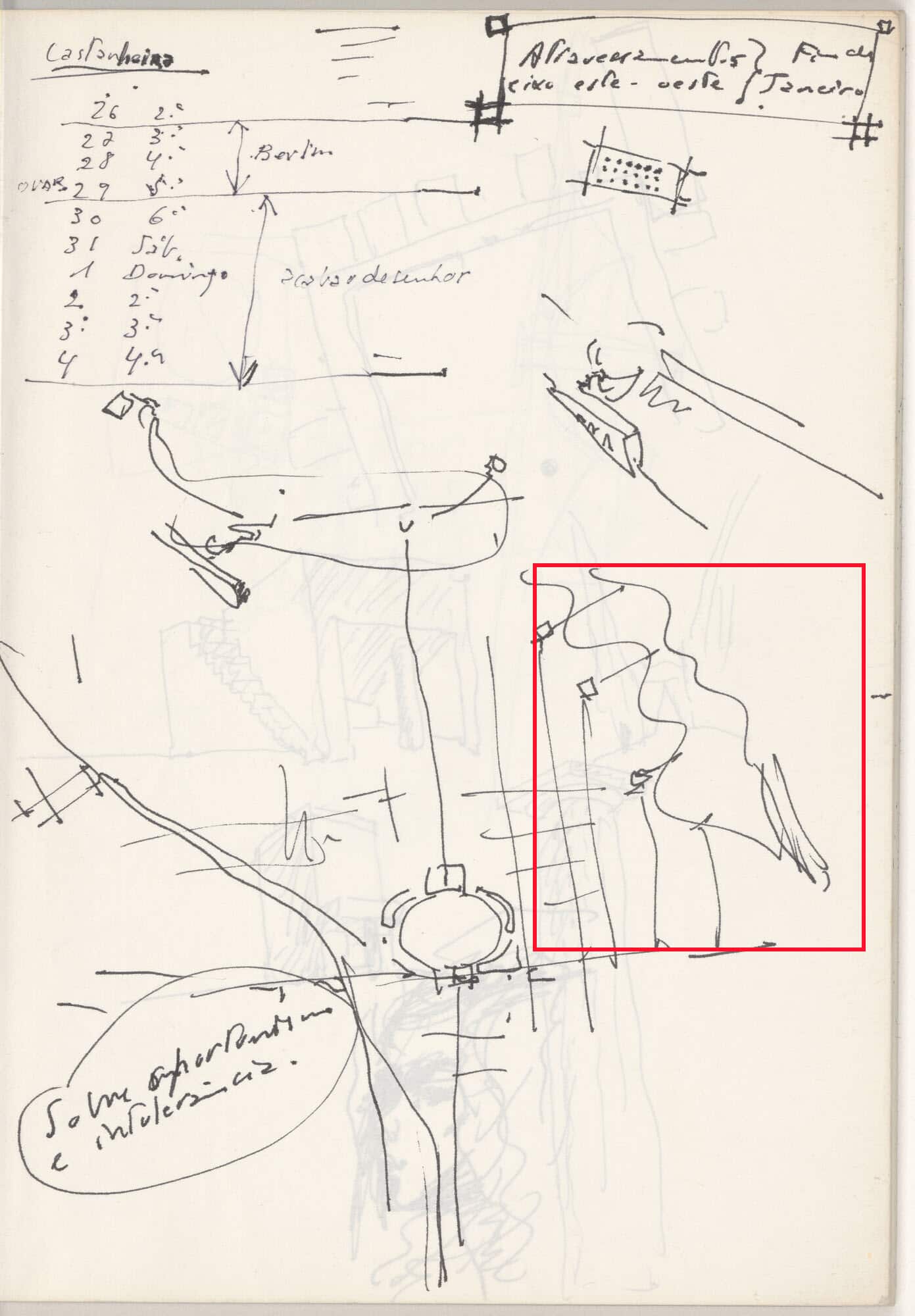
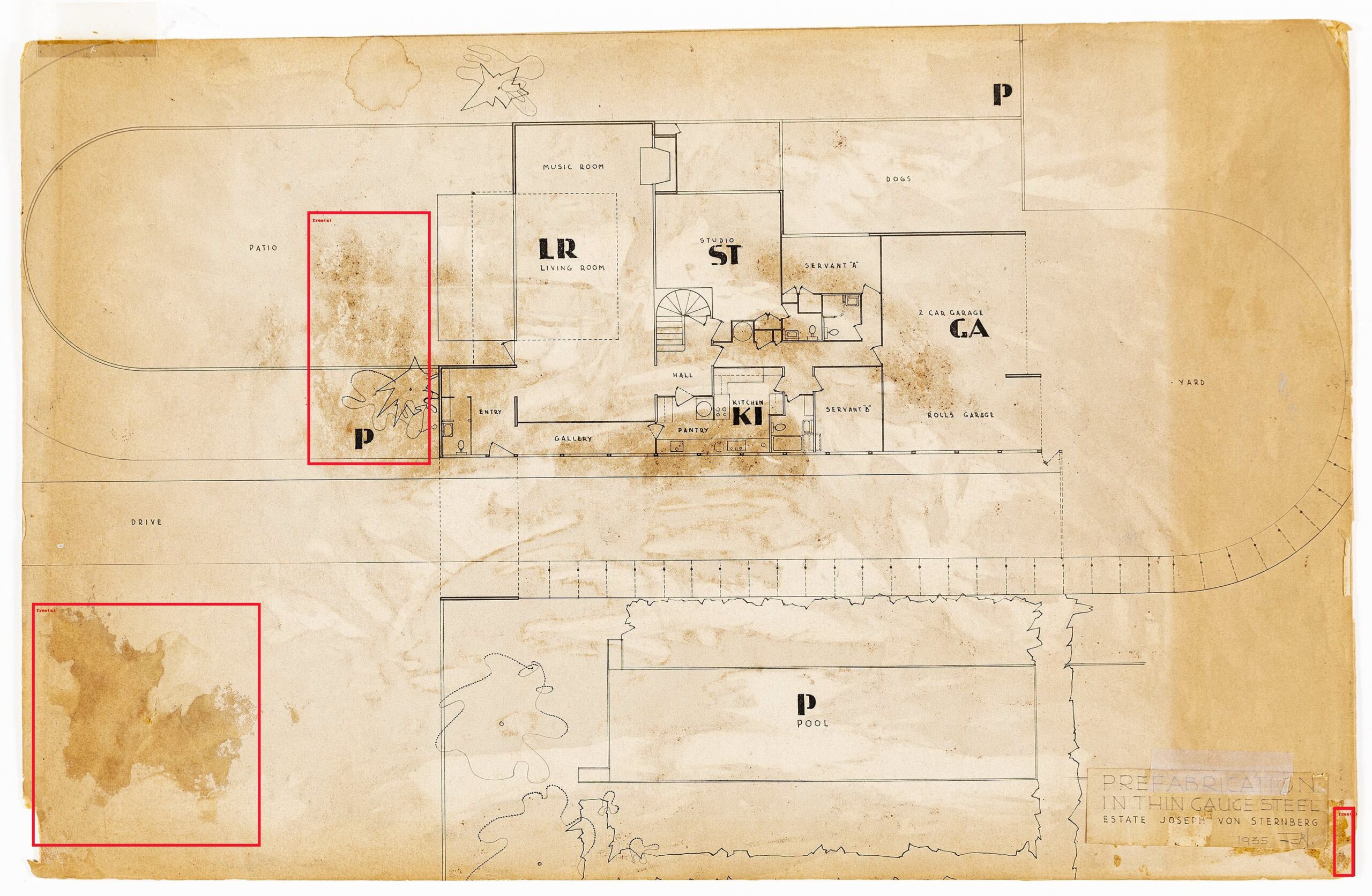
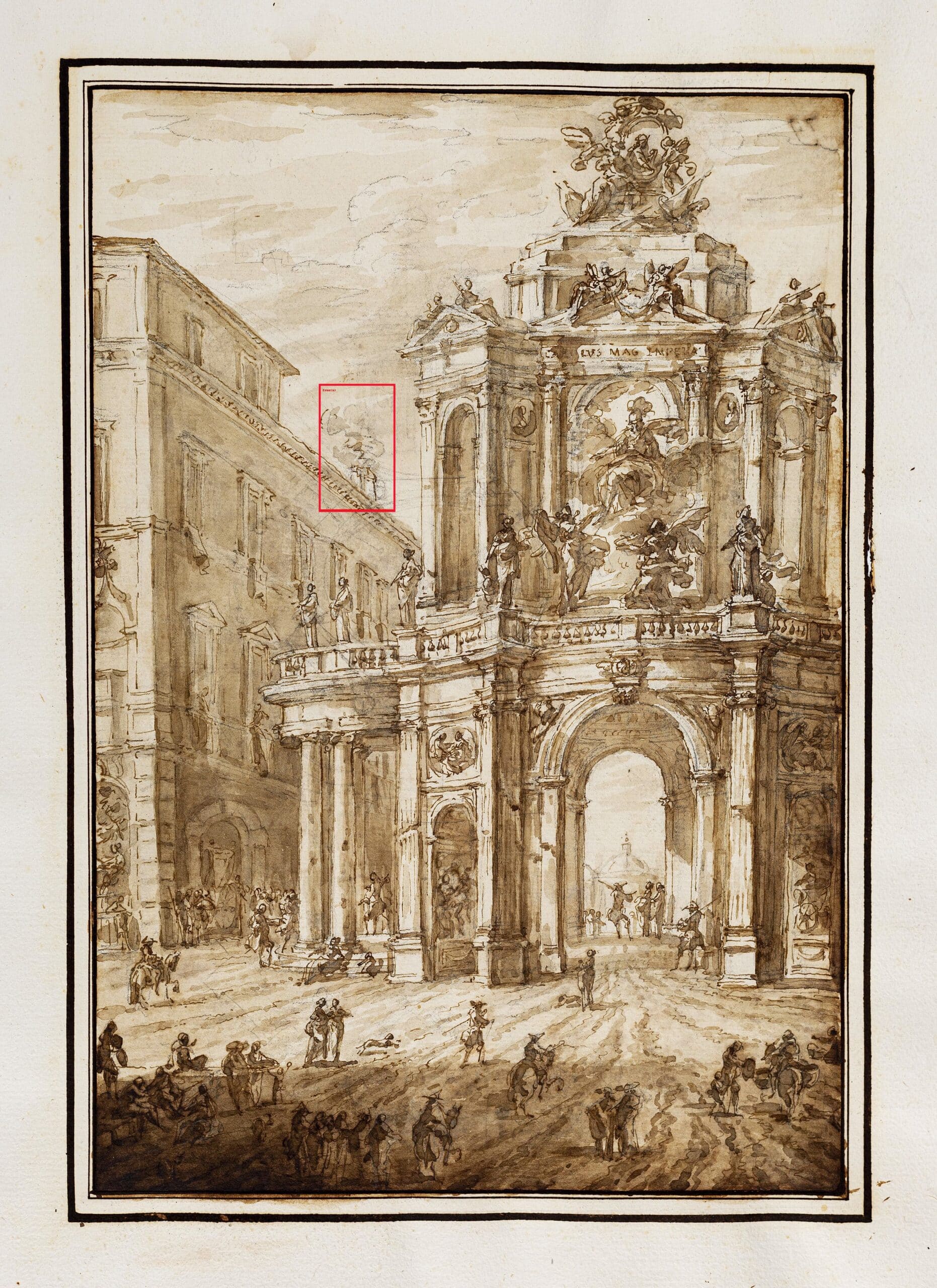
The tool learned well that architects often draw trees—whether in plan, section, elevation, or perspective—by focusing on the canopy and showing it as a rough and irregular circular outline. While the ubiquity of this graphic convention demonstrates a disregard for the particularities of tree species and may signal, within the history of architecture, a broader disregard for a whole range of environmental particularities, it also demonstrates the multiple ways in which organic matter and its patterns of growth live within the materials that serve as the substrate of the field. For example, the tool confused tree canopies and clouds because they are frequently drawn as virtually indistinguishable figures. This conventional graphic presence, furthermore, is reinforced by a parallel conventional graphic absence. The earth in which trees grow and the atmosphere in which clouds form are typically signaled in architectural drawings by nothingness, or rather by nothing other than context: they appear without material positivity, merely as the surface of the drawing’s material substrate. These standard architectural abstractions and forms of neglect, however, enabled the machine to continue to apply architecture’s theory of organic morphological patterns indiscriminately and without attention to contextual cues, until eventually it stumbled across traces of movements of other elemental media within the archive. For the tool, drawings of trees and clouds and the indexical residue of mould and water stains are all interchangeable, which in an environmental media theoretical sense, they in fact are.
Digital Storytelling
This story was written by ChatGPT in response to the following prompts in sequence: explain the difference between a real tree and a fake tree; write a story about an architect who thinks she is a tree; write a horror story about an architect who thinks she is a tree. The responses were edited for length.
As a young architect, Lily had always been fascinated by trees. She loved the way they towered over the world, reaching up towards the sky, and the way they seemed to embody a sense of calm and peace. But as she grew older, her fascination with trees turned into something darker, something more sinister. Lily began to spend more and more time in the park, sitting beneath the trees and listening to their whispers. She became convinced that she was not just an architect, but a tree herself, and she would often spend hours staring up at the branches, lost in her own thoughts.
But one day, something changed. As she sat beneath a tall, ancient oak tree, she felt a sharp pain shoot through her body. It was as if the tree had reached out and grabbed her, its roots digging into her skin and pulling her down into the earth.
Her skin took on a greenish tint, and her hands became gnarled and twisted, like the branches of a tree. Her fingers fused together, forming a rough bark-like texture, and her feet rooted themselves to the ground, making it impossible for her to move.
Lily could hear the whispers of the trees around her, their voices growing louder and more insistent with each passing day. They spoke to her in a language she didn’t understand, telling her secrets and urging her to do things she knew she shouldn’t. As the sun rose over the park the next morning, the trees around Lily swayed in the breeze, their branches reaching out like grasping fingers. And in their midst, a single figure stood, motionless and silent, her body twisted and transformed into something that was no longer quite human.
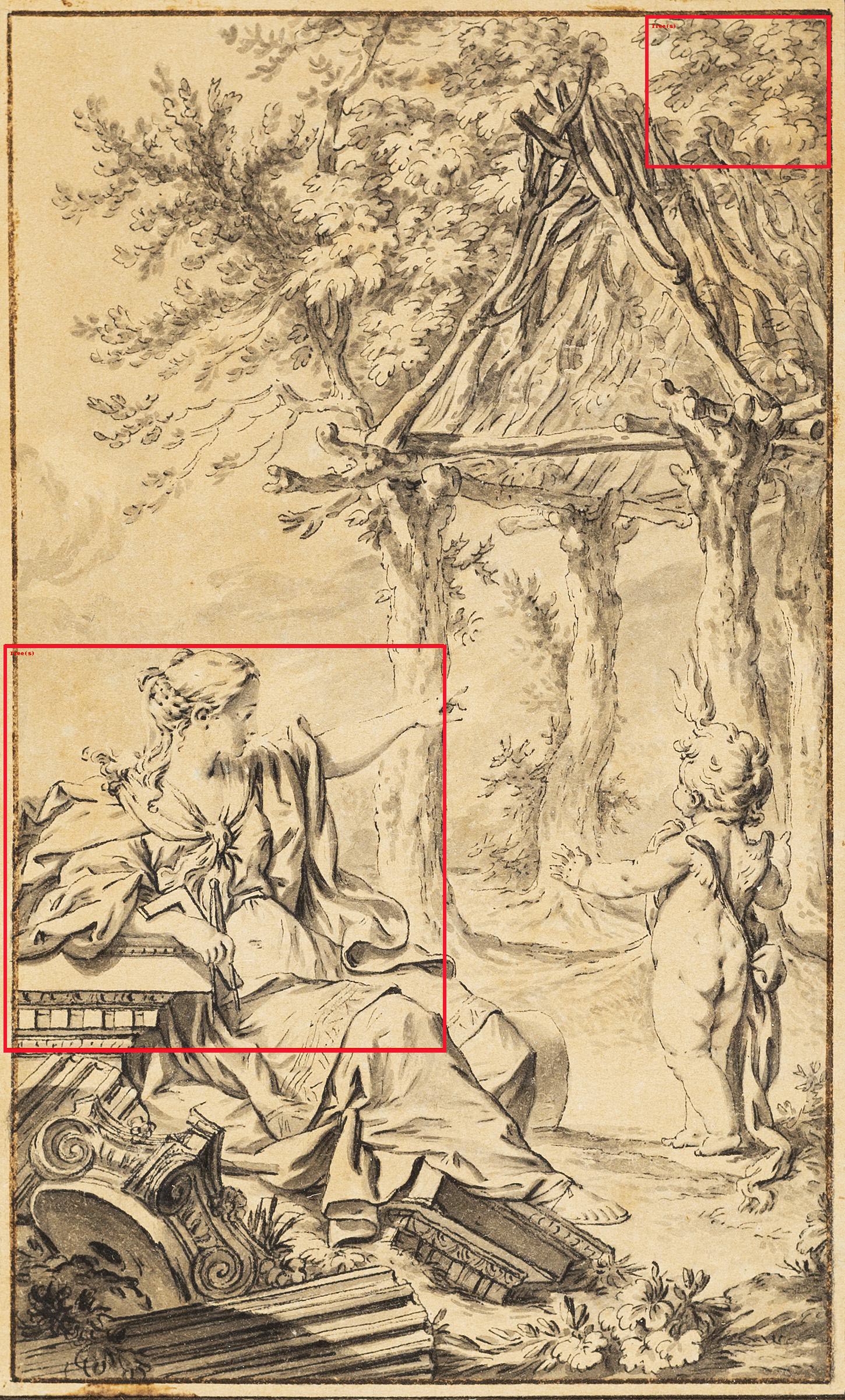
Notes
A special thanks to Elspeth Cowell (Head, Digital Media, CCA), Matt Page (Deputy Director of Drawing Matter) Zied Touzani and Emad Takla (Deloitte), and the following Princeton University students, Christina Moushoul (Project leader), Reese Greenlee, Joey Guadagno, Kajsa Souter, Ana Morris, and Sophia Solganik.
- Model training was done by data scientists at DataPerformers and Deloitte in Montreal.
- In some cases, documents are replacing drawings as preferred objects of study less as a means to critique the architectural cult of drawing but because of a false belief in their self-evident demonstrativeness. However naïve, the idea that documents intrinsically speak for themselves is reinforced by proliferating forms of accessibility that make documents apparently easier to read: legibility of documents is facilitated both by OCR software and by the idea that their material qualities are less important than those of drawing and hence their ‘meaning’ less degraded by digital reproduction.
- Of course, trees are commonly present in materials located through subject headings like garden or landscape, but the goal here was precisely to eschew such overdeterminations.
- This project followed established ML procedures: architecture students at Princeton were given preliminary training sets of digitized drawings on which they drew bounding boxes around trees as well as indicating the drawing projection. These materials were given to the data scientists at Dataperformers and Deloitte, who trained the data on a pre-trained object detection model using a CenterNet Resnet50 architecture. The results were reviewed by the scriptors and the CCA and false negatives and positives were identified and then used to refine the algorithm. This process was followed through three iterations, each with increasing number of drawings. The moment of hand off between algorithm and persons, themselves with varying area of expertise, is, like in any relay, the most vulnerable point in the process.
- In his Ordonnance for the Five Kinds of Columns after the Method of the Ancients, Claude Perrault famously identified symmetry as what he called an absolute element of beauty.
- Just to be sure, I asked ChatGPT “do you have an unconscious?” The response was, ‘as an artificial intelligence language model, I do not have consciousness or an unconscious mind. I am a machine that processes information according to programming and algorithms. My responses are generated based on patterns in data that I have been trained on, and I do not have subjective experiences or self-awareness.’
- The precise prompt for Google Bard chat was ‘why might a machine learning protocol confuse people and trees?’ The response was edited for length.